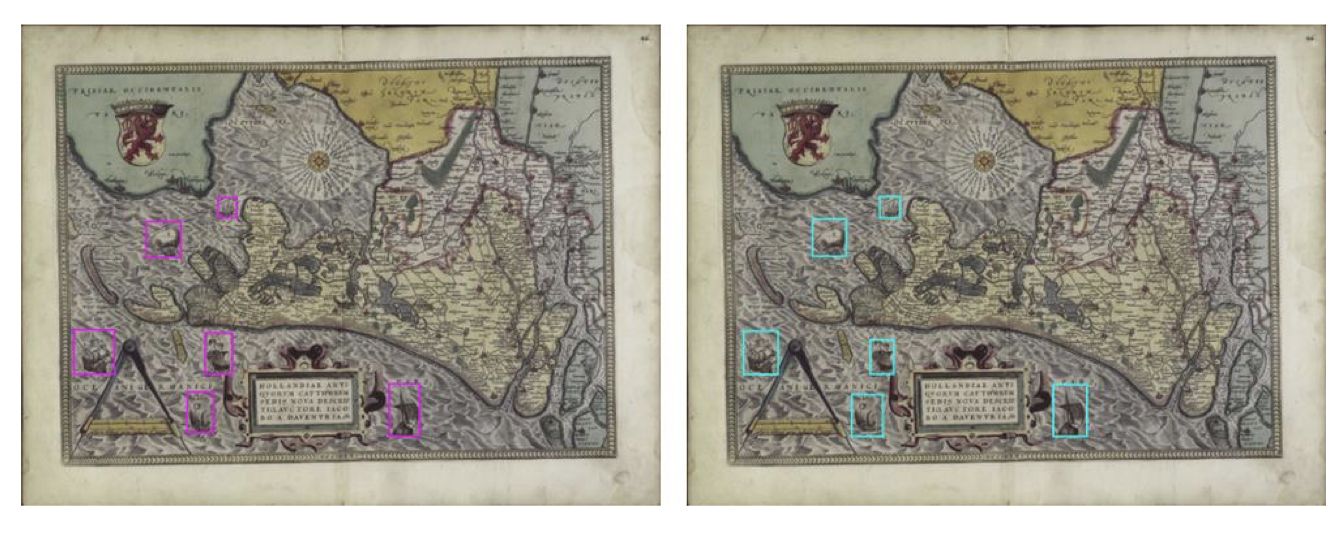
Raimund Schnürer1, René Sieber1, Jost Schmid-Lanter2, Cengiz Öztireli3, Lorenz Hurni1
1Institute of Cartography and Geoinformation, ETH Zurich, Zurich, Switzerland
2Abteilung Karten und Panoramen, Zentralbibliothek Zürich, Zurich, Switzerland
3Department of Computer Science and Technology, University of Cambridge, Cambridge, United Kingdom
In this work, realistically drawn objects are identified on digital maps by convolutional neural networks. For the first two experiments, 6200 images were retrieved from Pinterest. While alternating image input options, two binary classifiers based on Xception and InceptionResNetV2 were trained to separate maps and pictorial maps. Results showed that the accuracy is 95–97% to distinguish maps from other images, whereas maps with pictorial objects are correctly classified at rates of 87–92%. For a third experiment, bounding boxes of 3200 sailing ships were annotated in historic maps from different digital libraries. Faster R-CNN and RetinaNet were compared to determine the box coordinates, while adjusting anchor scales and examining configurations for small objects. A resulting average precision of 32% was obtained for Faster R-CNN and of 36% for RetinaNet. Research outcomes are relevant for trawling map images on the Internet and for enhancing the advanced search of digital map catalogues.
Links:
PDF Article Page