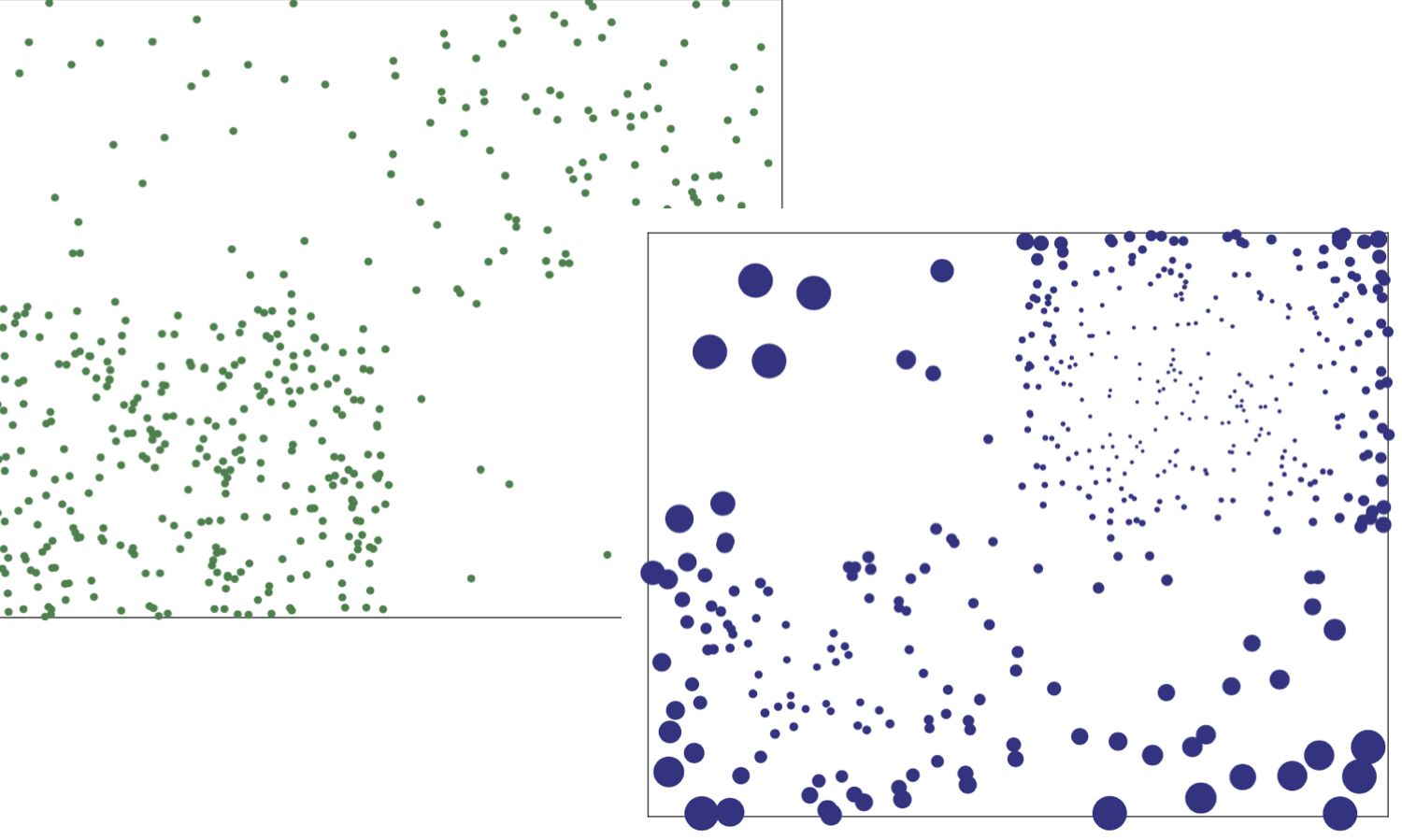
Cheng Zhang1, Cengiz Öztireli1, Stephan Mandt1
1Disney Research
Uniform mini-batch sampling may be beneficial in stochastic variational inference (SVI) and more generally in stochastic gradient descent (SGD). In particular, sampling data points with repulsive interactions, ie, suppressing the probability of similar data points in the same mini-batch, was shown to reduce the stochastic gradient noise, leading to faster convergence. When the data set is furthermore imbalanced, this procedure may have the additional benefit of sampling more uniformly in data space. In this work, we explore the benefits of using point processes with repulsive interactions for mini-batch selection. We thereby generalize our earlier work on using determinantal point processes for this task [14]. We generalize the proof of variance reduction and show that all we need is a point process with repulsive correlations. Furthermore, we investigate the popular Poisson disk sampling approach which is widely used in computer graphics, but not well known in machine learning. We finally show empirically that Poisson disk sampling achieves similar performance as determinantal point processes at a much more favorable scaling with the size of the dataset and the mini-batch size.
Links:
PDF