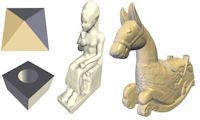
Cengiz Öztireli1, Gael Guennebaud2,3, Markus Gross1
1ETH Zurich, Switzerland 2National Research Council, Italy 3INRIA Bordeaux, France
Moving least squares (MLS) is a very attractive tool to design effective meshless surface representations. However, as long as approximations are performed in a least square sense, the resulting definitions remain sensitive to outliers, and smooth‐out small or sharp features. In this paper, we address these major issues, and present a novel point based surface definition combining the simplicity of implicit MLS surfaces [SOS04,Kol05] with the strength of robust statistics. To reach this new definition, we review MLS surfaces in terms of local kernel regression, opening the doors to a vast and well established literature from which we utilize robust kernel regression. Our novel representation can handle sparse sampling, generates a continuous surface better preserving fine details, and can naturally handle any kind of sharp features with controllable sharpness. Finally, it combines ease of implementation with other non-robust approaches.
Links:
PDF