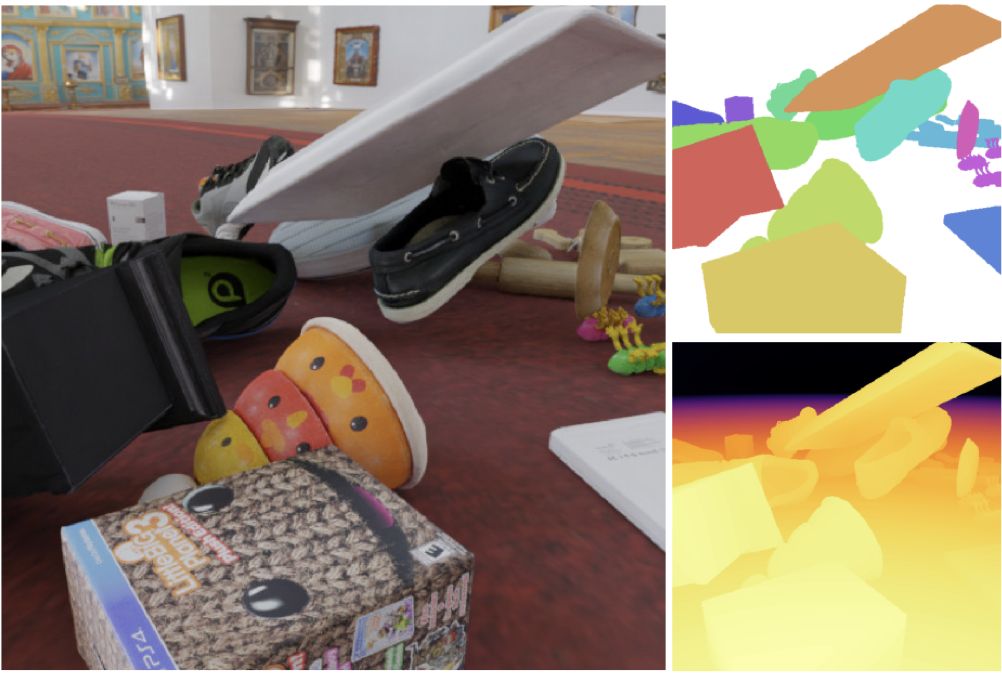
Klaus Greff1, Francois Belletti1, Lucas Beyer1, Carl Doersch6, Yilun Du5, Daniel Duckworth1, David J Fleet1,2, Dan Gnanapragasam1, Florian Golemo4,9, Charles Herrmann1, Thomas Kipf1, Abhijit Kundu1, Dmitry Lagun1, Issam Laradji3,9, Hsueh-Ti (Derek) Liu2, Henning Meyer1, Yishu Miao10, Derek Nowrouzezahrai3,4, Cengiz Öztireli1,8, Etienne Pot1, Mehdi S. M. Sajjadi1, Matan Sela1, Noha Radwan1, Daniel Rebain1,7, Sara Sabour1,2, Vincent Sitzmann5, Austin Stone1, Deqing Sun1, Suhani Vora1, Ziyu Wang10, Tianhao Wu8, Kwang Moo Yi7, Fangcheng Zhong8, Andrea Tagliasacchi1,2,11
1Google 2University of Toronto 3McGill University 4Mila 5MIT 6DeepMind
7UBC 8University of Cambridge 9ServiceNow 10Haiper 11Simon Fraser University
Data is the driving force of machine learning, with the amount and quality of training data often being more impor- tant for the performance of a system than architecture and training details. But collecting, processing and annotating real data at scale is difficult, expensive, and frequently raises additional privacy, fairness and legal concerns. Synthetic data is a powerful tool with the potential to address these shortcomings: 1) it is cheap 2) supports rich ground-truth annotations 3) offers full control over data and 4) can cir- cumvent or mitigate problems regarding bias, privacy and licensing. Unfortunately, software tools for effective data generation are less mature than those for architecture de- sign and training, which leads to fragmented generation efforts. To address these problems we introduce Kubric, an open-source Python framework that interfaces with PyBullet and Blender to generate photo-realistic scenes, with rich annotations, and seamlessly scales to large jobs distributed over thousands of machines, and generating TBs of data. We demonstrate the effectiveness of Kubric by presenting a series of 13 different generated datasets for tasks ranging from studying 3D NeRF models to optical flow estimation. We release Kubric, the used assets, all of the generation code, as well as the rendered datasets for reuse and modification.
Links:
Source code and dataset Paper