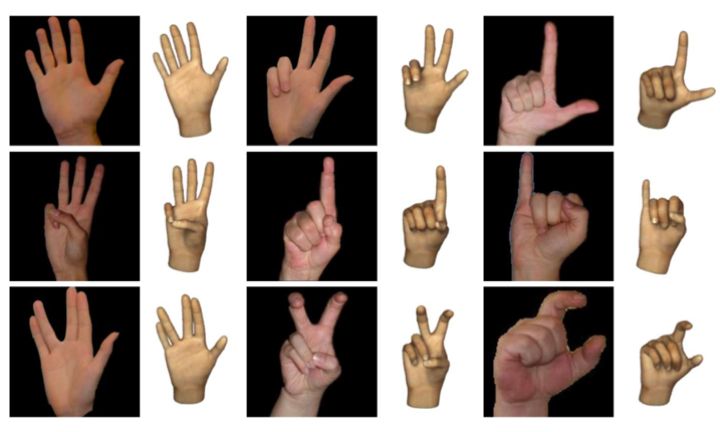
Endri Dibra, Silvan Melchior, Ali Balkis, Thomas Wolf, Cengiz Öztireli, Markus Gross
Department of Computer Science, ETH Zürich
3D hand pose inference from monocular RGB data is a challenging problem. CNN-based approaches have shown great promise in tackling this problem. However, such approaches are data-hungry, and obtaining real labeled training hand data is very hard. To overcome this, in this work, we propose a new, large, realistically rendered hand dataset and a neural network trained on it, with the ability to refine itself unsupervised on real unlabeled RGB images, given corresponding depth images. We benchmark and validate our method on existing and captured datasets, demonstrating that we strongly compare to or outperform state-of-the-art methods for various tasks ranging from 3D pose estimation to hand gesture recognition.
Links:
PDF Project page Supplementary