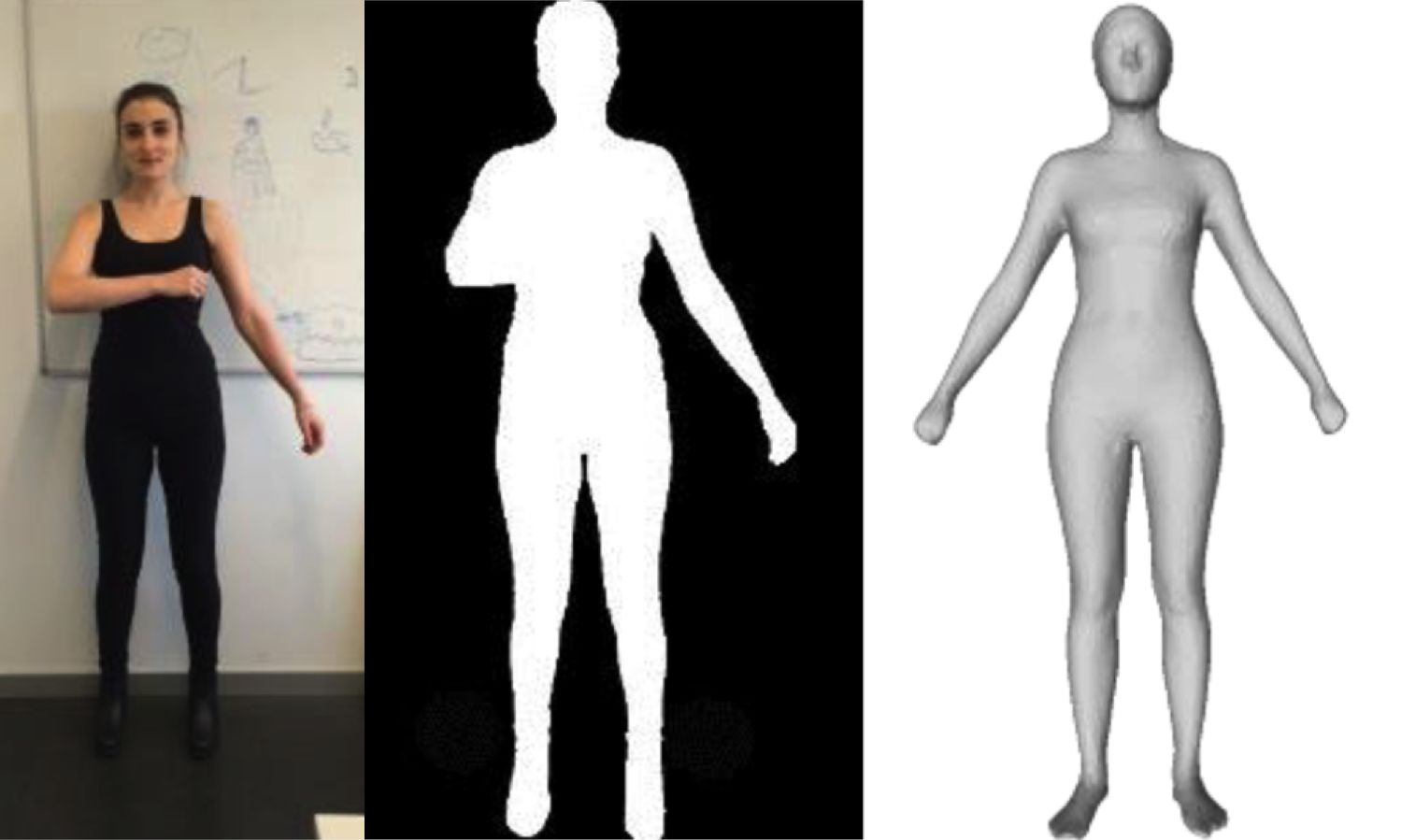
Endri Dibra1, Cengiz Öztireli1, Remo Ziegler2, Markus Gross1
1Department of Computer Science, ETH Zürich 2Vizrt
In this work, we revise the problem of human body shape estimation from monocular imagery. Starting from a statistical human shape model that describes a body shape with shape parameters, we describe a novel approach to automatically estimate these parameters from a single input shape silhouette using semi-supervised learning. By utilizing silhouette features that encode local and global properties ro- bust to noise, pose and view changes, and projecting them to lower di- mensional spaces obtained through multi-view learning with canonical correlation analysis, we show how regression forests can be used to com- pute an accurate mapping from the silhouette to the shape parameter space. This results in a very fast, robust and automatic system under mild self-occlusion assumptions. We extensively evaluate our method on thousands of synthetic and real data and compare it to the state-of-art approaches that operate under more restrictive assumptions.
Links:
PDF Project page Supplementary